Improved method to identify and reduce uncertainties in marine carbon cycle predictions
How well do contemporary Earth System Models (ESMs) represent the dynamics of the modern day ocean? Often we question the fidelity of biological and chemical processes represented in these ESMs. The fact is representations of biogeochemical processes in models are plagued with some degree of uncertainties; therefore, identifying and reducing such deficiencies could advance ESM development and improve model predictions.
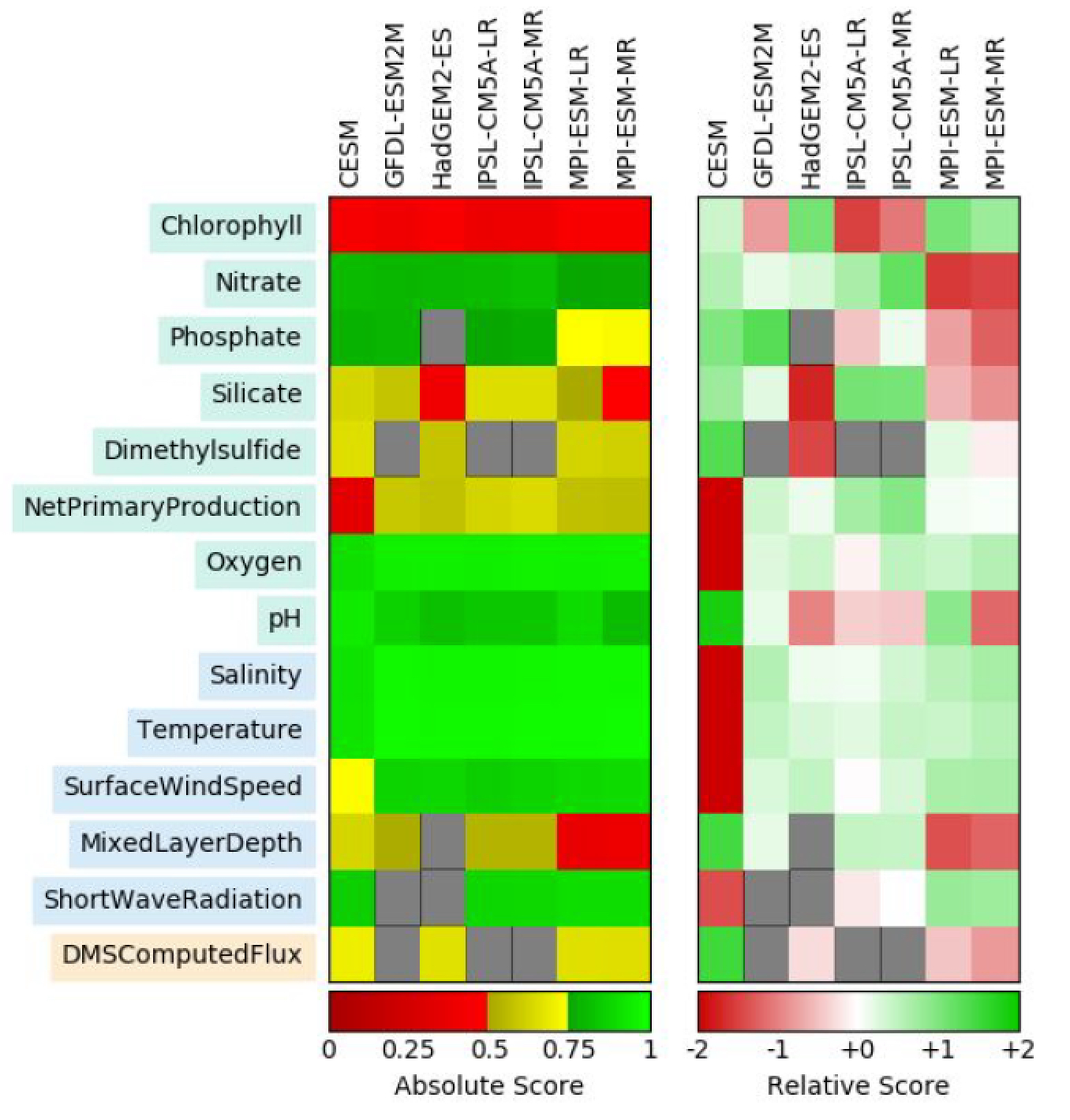
An overview of several models with respect to each of the variables, using absolute (left) and relative (right) scores to determine the degree of uncertainty in relation to referenced datasets.
A recent publication in Atmosphere described the ongoing efforts to develop the International Ocean Model Benchmarking (IOMB) package to evaluate ESM skill sets in simulating marine biogeochemical variables and processes. Model performances were scored based on how well they captured the distribution and variability contained in high-quality observational datasets. The authors highlighted systematic model–data benchmarking as a technique to identify ocean model deficiencies, which could provide a pathway to improving representations of sub-grid-scale parameterizations. They have scaled the absolute score from zero to unity, where the red color tends toward zero to quantify weaknesses in the skill set of a particular model in capturing values from the observational datasets. On the other side of the spectrum, the green color signifies considerable temporal and spatial overlap between the predicted and the observational values. The authors also present the standard score to show the relative scores within two standard deviations from the model mean. The benchmarking package was employed in the published study to assess marine biogeochemical process representations, with a focus on surface ocean concentrations and sea–air fluxes of dimethylsulfide (DMS). The production and emission of natural aerosols remain one of the major limitations in estimating global radiative forcing. Appropriate representation of aerosols in the marine boundary layer (MBL) is essential to reduce uncertainty and provide reliable information on offsets to global warming. Results show that model–data biases increased as DMS enters the MBL, with models over-predicting sea surface concentrations in the productive region of the eastern tropical Pacific by almost a factor of two and the sea–air fluxes by a factor of three. The associated uncertainties with oceanic carbon cycle processes may be additive or antagonistic; in any case, a constructive effort to disentangle the subtleties begins with an objective benchmarking effort, which is focused specifically on marine biogeochemical processes. The tool in development will ensure we satisfy some of the Model Intercomparison Project (MIP) benchmarking needs for the sixth phase of Coupled Model Intercomparison Project (CMIP6).
Authors:
Oluwaseun Ogunro (ORNL)
Scott Elliott (LANL)
Oliver Wingenter (New Mexico Tech)
Clara Deal (University of Alaska)
Weiwei Fu (UC Irvine)
Nathan Collier (ORNL)
Forrest M. Hoffman (ORNL)