The California Current Large Marine Ecosystem (CCLME) is a productive coastal ecosystem extending from Baja California, Mexico, to British Columbia, Canada. High primary productivity is sustained by inputs of cooler, nutrient-rich waters during seasonal wind-driven upwelling in spring and summer. This high productivity fuels higher trophic levels, including highly valued commercial ($3.5B yr-1) and recreational ($2.5B yr-1) US fisheries (NOAA 2016). The CCLME system experiences large interannual and decadal variability in ocean conditions in response to the El Niño-Southern Oscillation (ENSO) and extratropical climate modes such as the Pacific Decadal Oscillation and the North Pacific Gyre Oscillation (Di Lorenzo et al. 2013). ENSO events affect productivity of the CCLME ecosystem through atmospheric and oceanic pathways. In the former, El Niño triggers a decrease in equatorward winds (Alexander et al. 2002), reducing upwelling and nutrient inputs to coastal surface waters (Schwing et al. 2002; Jacox et al. this issue). In the latter, El Niño events propagate poleward from the equator via coastally trapped Kelvin waves, increasing the depth of the thermocline, and hence decreasing the nutrient concentration of upwelled source waters during El Niño events (Jacox et al. 2015; Jacox et al. this issue). Thus, CCLME productivity, forage fish dynamics, and habitat availability for top predators can vary substantially between years (Chavez et al. 2002; Di Lorenzo et al. 2013; Hazen et al. 2013; Lindegren et al. 2013), and there is increasing recognition of the need to incorporate seasonal forecasts of ocean conditions into management frameworks to improve fisheries management and industry decisions (Hobday et al. 2016; Tommasi et al. 2017a). We describe herein recent improvements in the seasonal prediction of ENSO and how these advances have translated to skillful forecasts of oceanic conditions in the CCLME. We conclude by offering remarks on the implications for ecological forecasting and improved management of living marine resources in the CCLME.
Seasonal ENSO predictions
ENSO is the dominant mode of seasonal climate variability, and while it is a tropical Pacific phenomenon, its effects extend over the entire Pacific basin and even globally. ENSO and its teleconnections influence rainfall, temperature, and extreme events such as flooding, droughts, and tropical cyclones (Zebiak et al. 2015). Because of the extensive societal impacts associated with ENSO, its prediction has been central to the development of today’s state-of–the-art seasonal climate prediction systems. The first attempts at ENSO prediction go back to the 1980s (Cane et al. 1986). Today, resulting from the development of an ENSO observing system located in the equatorial Pacific (McPhaden et al. 1998) and large improvements in our understanding of ENSO dynamics over the last two decades (Neelin et al. 1998; Latif et al. 1998; Chen and Cane 2008), prediction systems can, in general, skillfully predict ENSO up to about six months in advance (Tippett et al. 2012; Ludescher et al. 2014). While such skillful ENSO forecasts may also improve prediction of the extratropical ENSO response, intrinsic variability of the extratropical atmosphere and ocean, and the chaotic nature of weather, will limit extratropical prediction skill no matter how accurately the models—and observations initializing them—predict ENSO itself. ENSO operational forecasts from numerous climate modeling centers are made available in real-time from Columbia University’s International Research Institute for Climate and Society and NOAA’s Climate Prediction Center.
Given its global impact, ENSO provides much of the climate forecasting skill on seasonal timescales (Goddard et al. 2001). While weather is only predictable over a timescale of days (up to about two weeks) owing to the chaotic nature of the atmosphere (Lorenz 1963), predictions of seasonal-scale anomalies are possible because of the ability of global dynamical prediction systems to model atmosphere-ocean coupling processes and other atmosphere forcing factors, such as land and sea ice, which vary more slowly than the atmosphere (Goddard 2001). Low-frequency variations in sea surface temperature (SST), particularly in the tropics, can modulate the atmosphere (as is the case for ENSO), making some weather patterns more likely to occur over the next month or season. Therefore, the ability of the coupled global climate models to skillfully forecast the evolution of observed tropical SSTs, shifts the distribution of likely average weather over the next month or season may be, and allows for skillful prediction of seasonal climate anomalies.
While seasonal predictability is relatively high for SST due to the ocean’s large thermal inertia, assessments of SST predictability have largely been focused on ocean basin-scale modes of variability (e.g., ENSO), linked to regional rainfall and temperature patterns over land. However, recent work has demonstrated that seasonal SST predictions are also skillful in coastal ecosystems (Stock et al. 2015; Hervieux et al. 2017), and, as detailed in the next section, specifically for the CCLME (Jacox et al. 2017).
Seasonal climate predictions in the California Current Large Marine Ecosystem
Recent advances in ENSO prediction and global dynamical seasonal climate prediction systems have enabled skillful seasonal forecasts of SST anomalies in the CCLME after bias correcting the forecasts to remove model drift (Stock et al. 2015; Jacox et al. 2017; Hervieux et al. 2017). Skill of SST anomaly predictions produced by the National Oceanic and Atmospheric Administration (NOAA) North American Multi-Model Ensemble (NMME) is shown in Figure 1. Skill is evaluated through the anomaly correlation coefficient (ACC) between monthly SST anomalies from retrospective forecasts from 1982 to 2009 and observed SST anomalies. Forecasts are skillful (ACC > 0.6) across initialization months for lead times up to about four months (Figure 1). Persistence of the initialized SST anomalies provides much of the prediction skill at these short lead times (Stock et al. 2015; Jacox et al. 2017). Preexisting temperature anomalies at depth may also provide some predictability. Skillful forecasts of February, March, and April SST extend to lead times greater than six months (Figure 1; Stock et al. 2015; Jacox et al. 2017). This ridge of enhanced predictive skill in winter to early spring forecasts is apparent across seasonal forecasting models and arises from the ability of the prediction systems to capture the wintertime coastal signature of predictable basin-scale SST variations (Stock et al. 2015; Jacox et al. 2017). Specifically, the models can skillfully forecast the predictable evolution of meridional winds during ENSO events and the associated changes in upwelling anomalies and SST in the CCLME (Jacox et al. 2017).
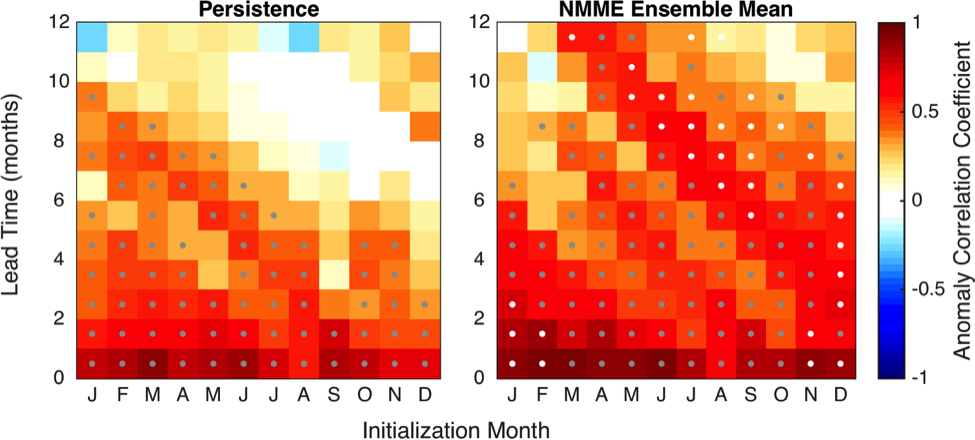
Figure 1. Anomaly correlation coefficients (ACCs) as a function of forecast initialization month (x-axis) and lead-time (y-axis) for (left) persistence and (right) NOAA NMME mean for the California Current system (US West Coast, less than 300 km from shore). Note the ridge of high SST anomaly prediction skill exceeding persistence at long lead-times (4-12 months) for late winter-early spring forecasts. Grey dots indicate ACCs significantly above zero at a 5% level; white dots indicate ACCs significantly above persistence at a 5% level. (Adapted from Jacox et al. 2017).
Owing to the severe ecological and economic consequences of extreme SST conditions in the CCLME (e.g., Cavole et al. 2016), it is also instructive to look at forecast performance over time, specifically during the CCLME extreme warm events of 1991-1992, 1997-1998, and 2014-2016, and the CCLME extreme cold events of 1988-1989, 1998-1999, and 2010-2011 (Figure 2). All of the cold events were associated with La Niña conditions, and the first two warm events and 2015-2016 were associated with El Niño. However, the anomalously warm conditions of 2014 and 2015, dubbed “the blob,” were caused by a resilient ridge of high pressure over the North American West Coast that suppressed storm activity and mixing, and allowed a build-up of heat in the upper ocean (Bond et al. 2015).
The forecast system is highly skillful at one-month lead times. It is also skillful at longer lead times of three and six months, as seen by the forecasted February to April SSTs following the 2010-2011 La Niña and the 2015-2016 El Niño (Figure 2). However, at these longer lead times, the forecast system was unable to capture the extreme magnitude of the warm “blob” anomalies during 2014 and 2015 (Figure 2). Also, while fall to winter conditions during the 1991-1992 El Niño and the late winter-early spring conditions following the 1997-1998 El Niño were forecasted with a six-month lead time, the prolonged warm conditions over the 1992 summer and the early transition to anomalously warm conditions during the summer of 1997 were not (Figure 2).
Transitions in and out of the 1991 and 1997 El Niño events were particularly unusual also at the Equator, with El Niño conditions developing late in 1991 and persisting well into the summer of 1992, and El Niño conditions appearing early in summer 1997 (see Figure 2 in Jacox et al. 2015). The spring predictability barrier for ENSO (i.e., a dip in forecast skill for forecasts initialized over the ENSO transition period of March-May; Tippet et al. 2012), as well as weaker teleconnections to the extratropics in summer, may partly explain the lower forecast skill for these El Niño events during summer and fall, and the poorer forecast performance in predicting the early transition to La Niña conditions in 1998-1999 and 2010-2011 (Figure 2).
The forecast system was also unable to predict the cooler conditions over the ENSO-neutral spring and summer of 1991 (Figure 2). The conditional predictability of CCLME winds and SST on ENSO implies that during ENSO-neutral conditions, such as in 1991 and 2014, forecasts of winds are not skillful and SST forecast skill is therefore limited to lead times up to about four months (Jacox et al. 2017). Thus, skillfulness of the seasonal predictions results from a complex interplay of factors that will require further study to identify the underlying mechanisms driving differing levels of robustness.
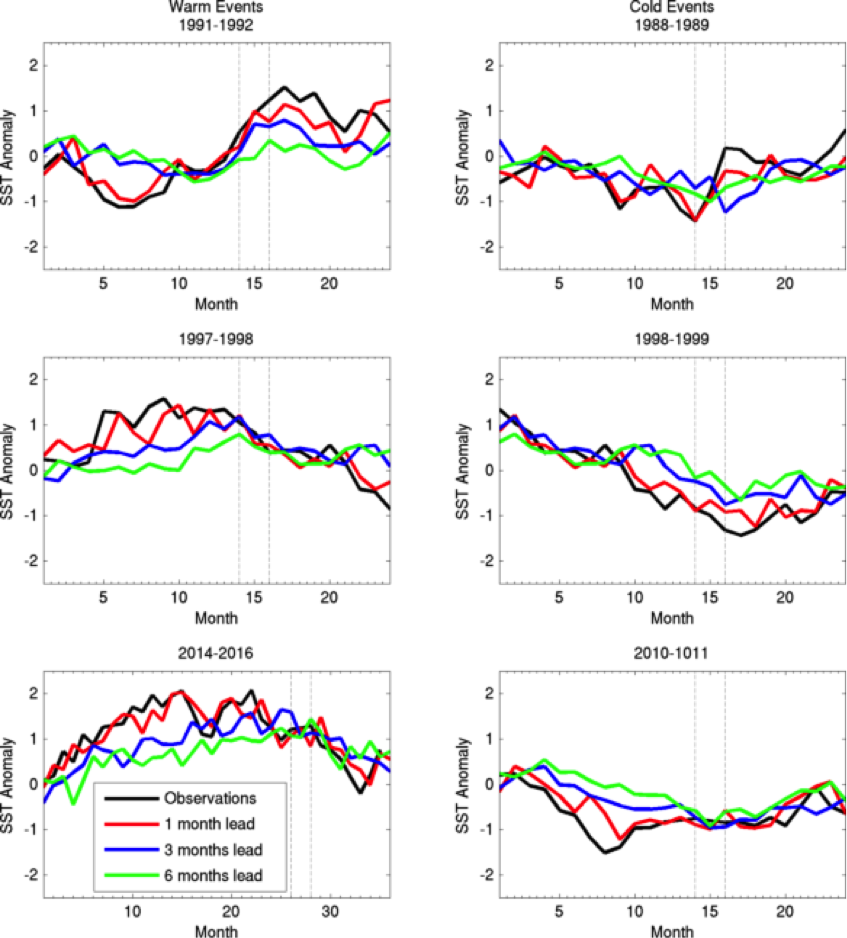
Figure 2. Predictions at 1-month (red line), 3-month (blue line), and 6-month (green line) lead times of SST anomalies (°C) for the CCLME from the NOAA Geophysical Fluid Dynamics Laboratory (GFDL) CM2.5 FLOR global climate prediction systems and Reynolds OISST.v2 observations (black line) for specific extreme events in the CCLME. Warm events are on the left; cold events are on the right. The dotted lines represent the February to April period of enhanced predictive skill following ENSO events. The x-axis is months since January 1 of the year in which the extreme event started.
Seasonal forecasts for fisheries management applications
While seasonal prediction of living marine resources has been a goal for the past three decades (GLOBEC 1997), operational use of seasonal SST forecasts to inform dynamic management of living marine resources was pioneered in Australia (Hobday et al. 2011), where seasonal SST forecasts are now used to improve the decision making of the aquaculture industry (Spillman and Hobday 2014; Spillman et al. 2015), fishers (Eveson et al. 2015), and fisheries managers (Hobday et al. 2011). Through both increased awareness of climate prediction skill at fishery-relevant scales and of their value to ecosystem-based management, such efforts have now begun to expand to other regions (see Tommasi et al. 2017a, and case studies therein). In the CCLME, recent work has demonstrated that integration of current March SST forecasts into fisheries models can provide useful information for catch limit decisions for the Pacific sardine fishery (i.e., how many sardines can be caught each year?) when combined with existing harvest cutoffs (Tommasi et al., 2017b). Knowledge of future SST conditions can improve predictions of future recruitment and stock biomass and allow for the development of a dynamic management framework, which could increase allowable fisheries harvests during periods of forecasted high productivity and reduce harvests during periods of low productivity (Tommasi et al. 2017b). Hence, integration of skillful seasonal forecasts into management decision strategies may contribute to greater long-term catches than those set by management decisions based solely on either past SST information or on no environmental information at all (Figure3; Tommasi et al., 2017b).
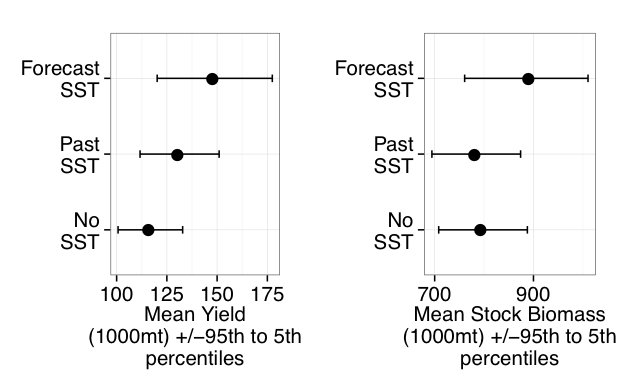
Figure 3. Mean long-term Pacific sardine catch and biomass following catch limit decisions integrating different levels of environmental information. The catch limit incorporating future SST information reflects the uncertainty of a 2-month lead forecast. (Adapted from Tommasi et al. 2017b).
Novel dynamical downscaling experiments in the Northern California Current as part of the JISAO Seasonal Coastal Ocean Prediction of the Ecosystem (J-SCOPE) project (Siedlecki et al. 2016) show that seasonal regional climate forecasts may also be of potential utility for dynamic spatial management strategies in the CCLME (Kaplan et al. 2016). Predictions of ocean conditions from a global dynamical climate prediction system (NOAA NCEP CFS) forced the Regional Ocean Modeling System (ROMS) with biogeochemistry to produce seasonal forecasts of ocean conditions, both at the surface and at depth, with measureable skill up to a four-month lead time (Siedlecki et al. 2016). The downscaling both enables forecasts of fishery-relevant biogeochemical variables such as chlorophyll, oxygen, and pH not yet produced by global forecasting systems, and resolves the fine-scale physical and ecological processes influencing the distribution of managed species within the CCLME. For instance, high-resolution regional implementations of ROMS resolve upwelling and coastal wave dynamics (Jacox et al. 2015; Siedlecki et al. 2016), two processes that drive the CCLME response to ENSO variability, better than coarser-resolution global models. Downscaled forecasts have also driven prototype forecasts of Pacific sardine spatial distribution (Kaplan et al. 2016). Such forecasts have the potential to inform fishing operations, fisheries surveys, and US and Canadian quotas for this internationally shared stock (Kaplan et al. 2016; Siedlecki et al. 2016; Tommasi et al. 2017a).
These CCLME case studies suggest that with recent advancements in state-of-the-art global dynamical prediction systems and regional downscaling models, some skillful seasonal predictions of ocean conditions are possible (Siedlecki et al. 2016; Tommasi et al. 2017a). Seasonal forecast skill may be further improved by improved representation of other features such as ocean eddies and gyre circulations in the extratropics and the basin-wide atmospheric response to SST anomalies in the Kuroshio-Oyashio region (Smirnov et al. 2015). Such skillful seasonal forecasts present opportunities for inclusion in adaptive management strategies for improved living marine resource management and better informed industry operations in the CCLME.
Authors
Desiree Tommasi (Princeton University)
Michael G. Jacox (University of California, Santa Cruz, NOAA Southwest Fisheries Science Center)
Michael A. Alexander Earth System Research Laboratory, NOAA
Francisco E. Werner (NOAA Southwest Fisheries Science Center)
Samantha Siedlecki (University of Washington)
Charles A. Stock Geophysical Fluid Dynamics Laboratory, NOAA
Nicholas A. Bond (University of Washington)
References
Alexander, M. A., I. Bladé, M. Newman, J. R. Lanzante, N. C. Lau, and J. D. Scott, 2002: The atmospheric bridge: The influence of ENSO teleconnections on air-sea interaction over the global oceans. J. Climate, 15, 2205-2231, doi: 10.1175/1520-0442(2002)015<2205:TABTIO>2.0.CO;2.
Bond, N. A., M. F. Cronin, H. Freeland, and N. Mantua, 2015: Causes and impacts of the 2014 warm anomaly in the NE Pacific. Geophys. Res. Lett., 42, 3414–3420, doi:10.1002/2015GL063306.
Cane, M. A., S. E. Zebiak, and S. C. Dolan, 1986: Experimental forecasts of El Niño. Nature 321, 827–832, doi:10.1038/321827a0.
Cavole, L. M., and Coauthors, 2016: Biological impacts of the 2013–2015 warm-water anomaly in the Northeast Pacific: Winners, losers, and the future. Oceanogr., 29, 273–285, doi: 10.5670/oceanog.2016.32.
Chavez, F. P., J. T. Pennington, C. G. Castro, J. P. Ryan, R. P. Michisaki, B. Schlining, P. Walz, K. R. Buck, A. McFadyen, and C. A. Collins, 2002: Biological and chemical consequences of the 1997–1998 El Niño in central California waters. Prog. Oceanogr., 54, 205–232, doi: 10.1016/S0079-6611(02)00050-2.
Chen, D., and M. A. Cane, 2008: El Niño prediction and predictability. J. Comp. Phys., 227, 3625-3640, doi: 10.1016/j.jcp.2007.05.014.
Di Lorenzo, E., and Coauthors, 2013: Synthesis of Pacific Ocean climate and ecosystem dynamics. Oceanogr. 26, 68–81, doi: 10.5670/oceanog.2013.76.
Eveson, J. P., A. J. Hobday, J. R. Hartog, C. M. Spillman, and K. M. Rough, 2015: Seasonal forecasting of tuna habitat in the Great Australian Bight. Fish. Res., 170, 39–49 doi: 10.1016/j.fishres.2015.05.008.
Global Ocean Ecosystem Dynamics (GLOBEC), 1997: Global Ocean Ecosystem Dynamics Science Plan. R. Harris and the members of the GLOBEC Scientific Steering Committee, Eds., IGBP secretariat, Stockholm, Sweden, 83 pp.
Goddard, L., S. J. Mason, S. E. Zebiak, C. F. Ropelewski, R. Basher, and M. A. Cane, 2001: Current approaches to seasonal-to-interannual climate predictions. Int. J. Climatology, 21, 1111–1152, doi: 10.1002/joc.636.
Hazen, E. L., and Coauthors, 2013: Predicted habitat shifts of Pacific top predators in a changing climate. Nat. Climate Change, 3, 234-238, doi:10.1038/nclimate1686.
Hervieux, G., M. Alexander, C. Stock, M. Jacox, K. Pegion, and D. Tommasi, 2017: Seasonal sea surface temperature anomaly prediction skill for coastal ecosystems using the North American multi-model ensemble (NMME). Climate Dyn., submitted.
Hobday, A. J., J. R. Hartog, C. M. Spillman, and O. Alves, 2011: Seasonal forecasting of tuna habitat for dynamic spatial management. Can. J. Fish. Aq. Sci., 68, 898-911, doi: 10.1139/f2011-031.
Hobday, A. J., C. M. Spillman, J. P. Eveson, and J. R. Hartog, 2016: Seasonal forecasting for decision support in marine fisheries and aquaculture. Fish.,. Oceanogr., 25, 45-56, doi: 10.1111/fog.12083.
Jacox, M. G., J. Fiechter, A. M. Moore, and C. A. Edwards, 2015: ENSO and the California Current coastal upwelling response. J. Geophys. Res. Oceans, 120, 1691–1702, doi:10.1002/ 2014JC010650.
Jacox, M., E. L. Hazen, K. D. Zaba, D. L. Rudnick, C. A. Edwards, A. M. Moore, and S. J. Bograd, 2016: Impacts of the 2015–2016 El Niño on the California Current System: Early assessment and comparison to past events. Geophys. Res. Lett. 43, 7072-7080, doi:10.1002/2016GL069716.
Jacox, M. G., M. A. Alexander, C. A. Stock, and G. Hervieux, 2017: On the skill of seasonal sea surface temperature forecasts in the California Current System and its connection to ENSO variability. Climate Dyn., in review.
Kaplan, I. C., G. D. Williams, N. A. Bond, A. J. Hermann, and S. Siedlecki, 2016: Cloudy with a chance of sardines: forecasting sardine distributions using regional climate models. Fish. Oceanogr., 25, 15–27, doi: 10.1111/fog.12131.
Latif, M., A. Sterl, E. Maier-Reimer, and M. M. Junge, 1998: Climate variability in a coupled GCM. Part I: the tropical Pacific. J. Climate, 6, 5–21, doi: 10.1175/1520-0442(1993)006<0005:CVIACG>2.0.CO;2.
Lindegren, M., D. M. Checkley Jr., T. Rouyer, A. D. MacCall, and N. C. Stenseth, 2013: Climate, fishing, and fluctuations of sardine and anchovy in the California Current. Proc. Nat. Acad. Sci., 110, 13672-13677, doi: 10.1073/pnas.1305733110.
Lorenz, E. N. 1963: Deterministic nonperiodic flow. J. Atmos. Sci., 20, 130-141, doi: 10.1175/1520-0469(1963)020<0130:DNF>2.0.CO;2.
Ludescher, J., A. Gozolchiani, M. Bogachev, A. Bunde, S. Havlin, and H. J. Schellnhuber, 2014: Very early warning of next El Niño. Proc. Nat. Acad. Sci., 111, 2064-2066, doi: 10.1073/pnas.1323058111.
McPhaden, M. J., and Coauthors, 1998: The TOGA observing system: a decade of progress. J. Geophys. Res., 103, 14,169-14,240, doi: 10.1029/97JC02906.
Neelin, J. D., D. S. Battisti, A. C. Hirst, F. F. Jin, Y. Wakata, T. Yamagata, and S. E. Zebiak, 1998: ENSO theory. J. Geophys. Res., 103, 14261–14290, doi: 10.1029/97JC03424.
NOAA, 2016: National Marine Fisheries Service Seafood Industry Impacts tool. https://www.st.nmfs.noaa.gov/apex/f?p=160:1.
Schwing, F. B., T. Murphree, L. DeWitt, and P. M. Green, 2002: The evolution of oceanic and atmospheric anomalies in the northeast Pacific during the El Niño and La Niña events of 1995–2001. Prog. Oceanogr., 54, 459–491, doi:10.1016/S0079-6611(02)00064-2.
Siedlecki, S. A., I. C. Kaplan, A. J. Hermann, T. T. Nguyen, N. A. Bond, J. A. Newton, G. D. Williams, W. T. Peterson, S. R. Alin, and R. A. Feely, 2016: Experiments with seasonal forecasts of ocean conditions for the northern region of the California Current upwelling system. Sci. Rep., 6, 27203, doi: 10.1038/srep27203.
Smirnov, D., M. Newman, M. A. Alexander, Y.-O. Kwon, and C. Frankignoul, 2015: Investigating the local atmospheric response to a realistic shift in the Oyashio sea surface temperature front. J. Climate, 28, 1126-1147, doi: 10.1175/JCLI-D-14-00285.1.
Spillman, C. M., J. R. Hartog, A. J. Hobday, and D. Hudson, 2015: Predicting environmental drivers for prawn aquaculture production to aid improved farm management. Aquaculture, 447, 56–65, doi: 10.1016/j.aquaculture.2015.02.008.
Spillman, C. M., and A. J. Hobday, 2014: Dynamical seasonal ocean forecasts to aid salmon farm management in a climate hotspot. Climate Risk Management, 1, 25-38, doi: 10.1016/j.crm.2013.12.001.
Stock, C. A., K. Pegion, G. A. Vecchi, M. A. Alexander, D. Tommasi, N. A. Bond, P. S. Fratantoni, R. G. Gudgel, T. Kristiansen, T. D. O’Brien, Y. Xue, and X. Yang, 2015: Seasonal sea surface temperature anomaly prediction for coastal ecosystems. Prog. Oceanogr., 137, 219-236, doi: 10.1016/j.pocean.2015.06.007.
Tippett, M. K., A. G. Barnston, and S. Li, 2012: Performance of recent multi-model ENSO forecasts. J. App. Meteorol. Climatol., 51, 637–654, doi: 10.1175/JAMC-D-11-093.1.
Tommasi, D., and Coauthors, 2017a. Managing living marine resources in a dynamic environment: the role of seasonal to decadal climate forecasts. Prog. Oceanogr., doi: 10.1016/j.pocean.2016.12.011.
Tommasi, D., C. Stock, K. Pegion, G. A. Vecchi, R. D. Methot, M. Alexander, and D. Checkley, 2017b: Improved management of small pelagic fisheries through seasonal climate prediction. Ecol. App., doi: 10.1002/eap.1458.
Zebiak, S. E., B. Orlove, A. G. Muñoz, C. Vaughan, J. Hansen, T. Troy, M. C. Thomson, A. Lustig, and S. Garvin, 2015: Investigating El Niño-Southern Oscillation and society relationships. WIREs Climate Change, 6, 17–34, doi: 10.1002/wcc.294.